期刊Frontiers in Human Neuroscience发表论文:基于自然功能磁共振成像对大脑时空模式细粒度解释的混合学习框架
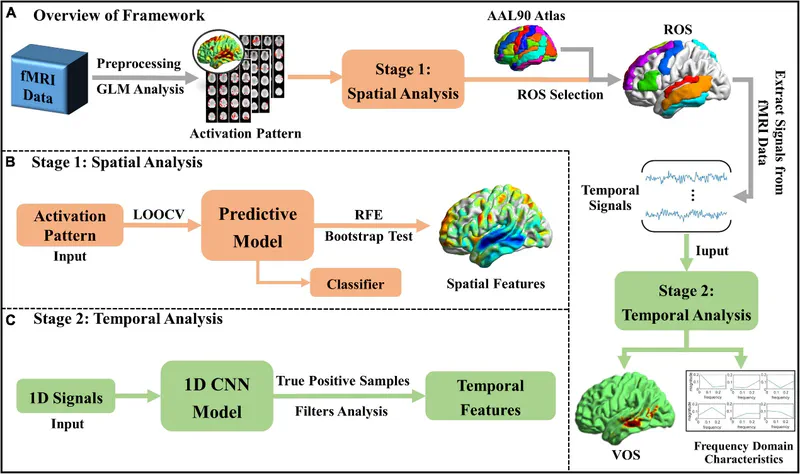
论文“A hybrid learning framework for fine-grained interpretation of brain spatiotemporal patterns during naturalistic functional magnetic resonance imaging”被期刊Frontiers in Human Neuroscience接收
时间:2022-09-30
关键词:脑影像人工智能,文章接收
近日,博士研究生喻四刚在神经科学领域期刊(Frontiers in Human Neuroscience,IF = 2.9,JCR Q2)发表题为“A hybrid learning framework for fine-grained interpretation of brain spatiotemporal patterns during naturalistic functional magnetic resonance imaging”的研究性文章,通讯作者为张枢教授。
Naturalistic stimuli, including movie, music, and speech, have been increasingly applied in the research of neuroimaging. Relative to a resting-state or single-task state, naturalistic stimuli can evoke more intense brain activities and have been proved to possess higher test–retest reliability, suggesting greater potential to study adaptive human brain function. In the current research, naturalistic functional magnetic resonance imaging (N-fMRI) has been a powerful tool to record brain states under naturalistic stimuli, and many efforts have been devoted to study the high-level semantic features from spatial or temporal representations via N-fMRI. However, integrating both spatial and temporal characteristics of brain activities for better interpreting the patterns under naturalistic stimuli is still underexplored. In this work, a novel hybrid learning framework that comprehensively investigates both the spatial (via Predictive Model) and the temporal [via convolutional neural network (CNN) model] characteristics of the brain is proposed. Specifically, to focus on certain relevant regions from the whole brain, regions of significance (ROS), which contain common spatial activation characteristics across individuals, are selected via the Predictive Model. Further, voxels of significance (VOS), whose signals contain significant temporal characteristics under naturalistic stimuli, are interpreted via one-dimensional CNN (1D-CNN) model. In this article, our proposed framework is applied onto the N-fMRI data during naturalistic classical/pop/speech audios stimuli. The promising performance is achieved via the Predictive Model to differentiate the different audio categories. Especially for distinguishing the classic and speech audios, the accuracy of classification is up to 92%. Moreover, spatial ROS and VOS are effectively obtained. Besides, temporal characteristics of the high-level semantic features are investigated on the frequency domain via convolution kernels of 1D-CNN model, and we effectively bridge the “semantic gap” between high-level semantic features of N-fMRI and low-level acoustic features of naturalistic audios in the frequency domain. Our results provide novel insights on characterizing spatiotemporal patterns of brain activities via N-fMRI and effectively explore the high-level semantic features under naturalistic stimuli, which will further benefit the understanding of the brain working mechanism and the advance of naturalistic stimuli clinical application.
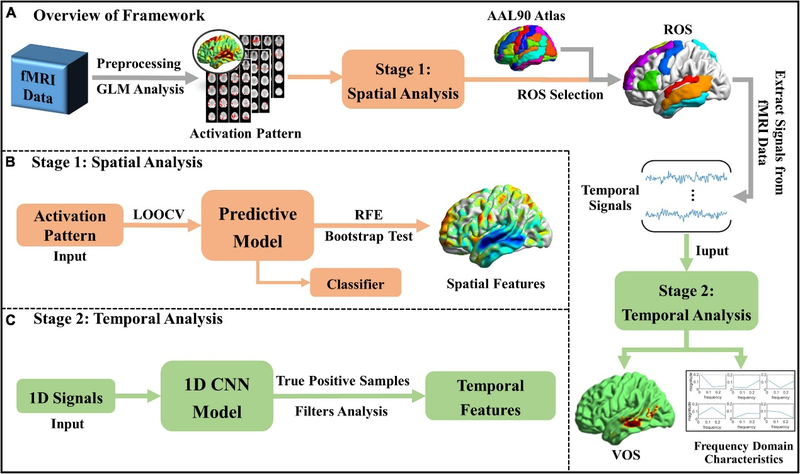
参考文献
Sigang Yu, Enze Shi, Ruoyang Wang, Shijie Zhao, Tianming Liu, Xi Jiang, and Shu Zhang. “A hybrid learning framework for fine-grained interpretation of brain spatiotemporal patterns during naturalistic functional magnetic resonance imaging.” Frontiers in Human Neuroscience 16, (2022): 944543.