会议BIBM accept regular论文:一种基于平滑条件域对抗训练的脑电运动想象解码框架
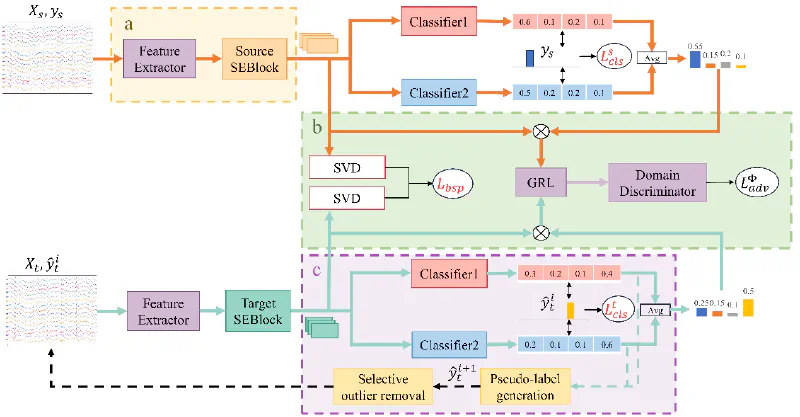
论文“A Smooth Conditional Domain Adversarial Training Framework for EEG Motor Imagery Decoding”被会议BIBM2024接受
时间:2024-08-16
关键词:脑机接口与智能机器人,文章接收
近日,硕士研究生袁麒龙在BIBM2024 上发表题为“A Smooth Conditional Domain Adversarial Training Framework for EEG Motor Imagery Decoding”的文章,通讯作者为张枢教授。
Objective:
The brain-computer interface (BCI) based on electroencephalogram (EEG) motor imagery (MI) decoding demonstrates promising application potential. However, the domain shift between training and testing data significantly impacts the model’s decoding efficacy. Domain adaption (DA) has been developed to address this problem recently. Nevertheless, existing DA methods have two limitations. One is that the extracted features are noisy, and the other is that they only align the distribution of features, which leads to limited generalization ability of the model. In this paper, we propose a novel smooth conditional domain adversarial training framework for solving the motor imagery decoding problem under domain shift. The framework uses interactive frequency convolution and channel attention mechanism as feature extractors to obtain effective features, and integrates smooth conditional domain adversarial training with batch spectral penalty to align the joint distribution of features and classes. At the same time, self-iterative training is implemented by generating pseudo-labels and selective outlier removal. Experimental results demonstrate that our proposed framework achieves 80.67% and 86.17% average accuracy in the BCI IV 2a and 2b respectively for cross-session experiments, achieving the best results compared with other methods, proving that the framework can improve the classification ability on the target domain while transferring effective features.
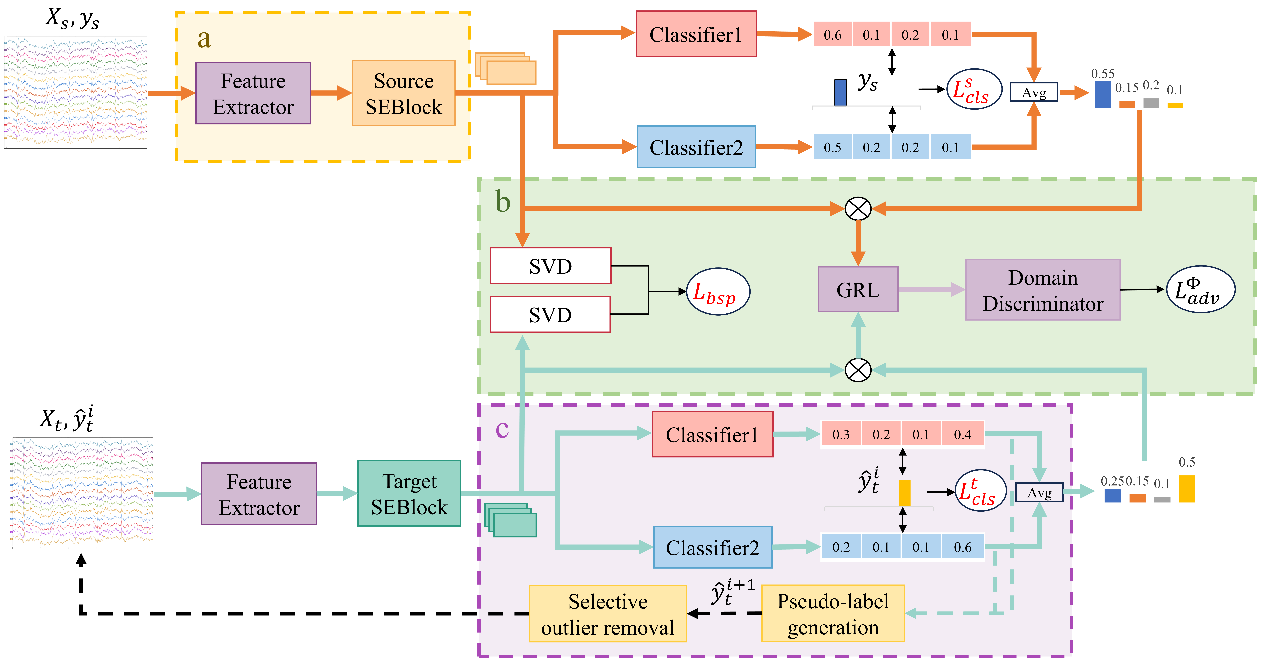
