会议MMMI发表论文:用于区分小型病理数据集的良恶性病变的基于袋集策略的多尺度纹理 GLCM-CNN 模型
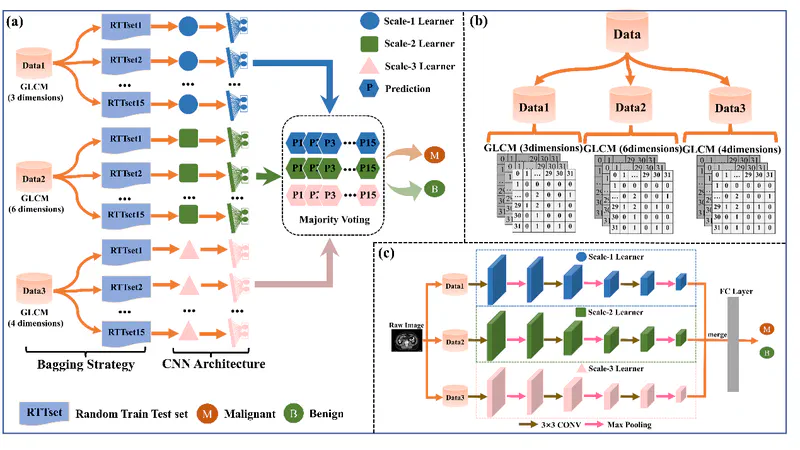
论文“A Bagging Strategy-Based Multi-scale Texture GLCM-CNN Model for Differentiating Malignant from Benign Lesions Using Small Pathologically Proven Dataset”被Multiscale Multimodal Medical Imaging接收
时间:2022-10-12
关键词:计算机辅助诊断,文章接收
近日,硕士研究生武晋茹在计算机科学领域会议(Multiscale Multimodal Medical Imaging)发表题为“A Bagging Strategy-Based Multi-scale Texture GLCM-CNN Model for Differentiating Malignant from Benign Lesions Using Small Pathologically Proven Dataset”的研究性文章,通讯作者为张枢教授。
The application of deep learning (DL) methodology in the differentiation of benign and malignant lesions has drawn wide attention. However, it is extremely hard to acquire medical images with biopsy labeling, which leads to the scarcity of datasets. This is contrary to the requirement that DL algorithms need large datasets for training. To effectively learn features from small tumor datasets, a Bagging Strategy-based Multi-scale gray-level co-occurrence matrix (GLCM)-Convolutional Neural Network (BSM-GLCM-CNN) is proposed to boost the classification performance. Specifically, instead of feeding the raw image to the CNN, GLCM is used as the input of the designed model. As a texture descriptor, GLCM has the advantages of effectively representing lesion heterogeneity and of the same size for all input samples given the gray level. This work creatively partitions the GLCM to three groups to make full use of certain scale information of each group. When fusing the multi-scale texture information, the concept of bagging strategy in ensemble learning is used to improve the classification performance, where multiple base Learners are generated. Final classification results are obtained by integrating the multi-scale base Learners with the voting mechanism. Experimental results show that the proposed BSM-GLCM-CNN can successfully distinguish colonic polyps in a small dataset. The proposed method achieves an improvement from 68.00% Area Under Curve (AUC) to 90.88% AUC over other state-of-the-art models. The experimental results demonstrate the great potential of the proposed method when challenged by small pathological datasets in the medical imaging field.
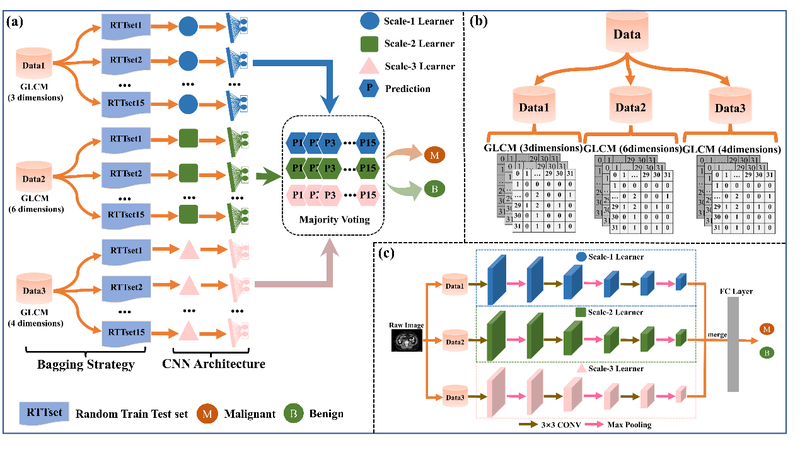
参考文献
Tan, J., Pickhardt, P.J., Gao, Y., et al.: 3D-GLCM CNN: a 3-dimensional gray-level co-occurrence matrix based CNN model for polyp classification via CT colonography. IEEE Trans. Med. Imaging PP(99), 1 (2019)