项目成果展示
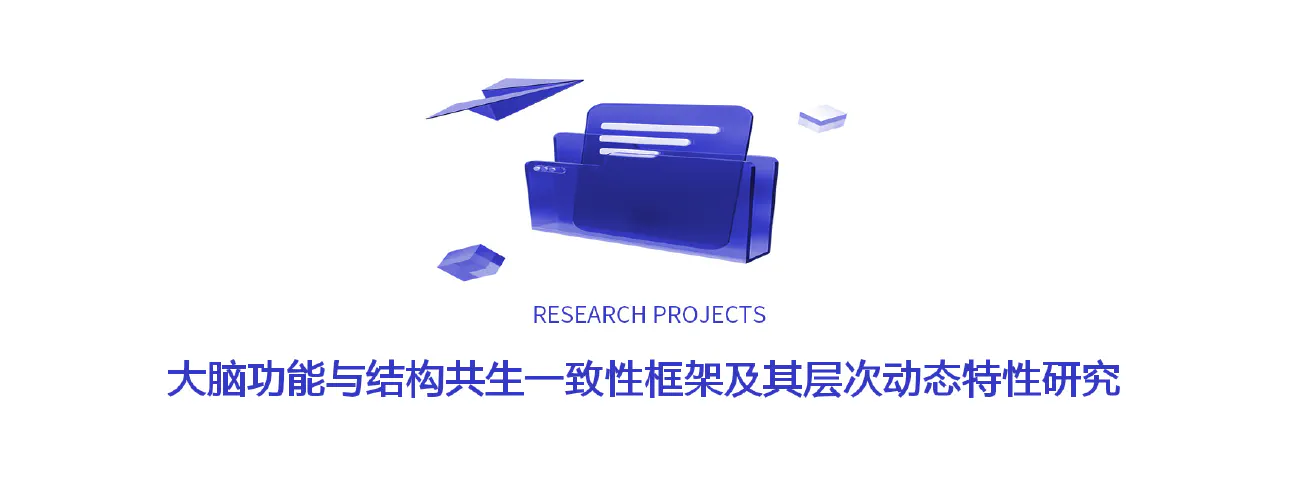
2023
2022
2021
2023年
期刊TBME发表论文:一种用于大脑状态解码的多频段EEG Transformer
Shi E, Yu S, Kang Y, et al. MEET:A Multi-Band EEG Transformer for Brain States Decoding[J]. IEEE Transactions on Biomedical Engineering, 2023.
https://ieeexplore.ieee.org/abstract/document/10345766
申请专利二:一种用于 EEG 通道筛选的自适应子图生成模型构建方法
顶会MICCAI 2023发表论文:基于自监督Graph-Transformer探索大脑功能结构连接体骨架
Kang Y, Wang R, Shi E, et al. Exploring Brain Function-Structure Connectome Skeleton via Self-supervised Graph-Transformer Approach[C]//International Conference on Medical Image Computing and Computer-Assisted Intervention. Cham:Springer Nature Switzerland, 2023:308-317.
https://link.springer.com/chapter/10.1007/978-3-031-43993-3_30
顶会MICCAI 2023发表论文:用于识别共同和一致的三铰链脑回的功能和结构特征联合表示
Zhang S, Wang R, Kang Y, et al. Joint Representation of Functional and Structural Profiles for Identifying Common and Consistent 3-Hinge Gyral Folding Landmark[C]//International Conference on Medical Image Computing and Computer-Assisted Intervention. Cham:Springer Nature Switzerland, 2023:163-172.
https://link.springer.com/chapter/10.1007/978-3-031-43993-3_16
会议ISBI发表论文:通过强化学习方法探索大脑连接中枢的新型多模态框架
Zhang S, Zhang H, Wang R, et al. A Novel Multi-Modality Framework for Exploring Brain Connectivity Hubs Via Reinforcement Learning Approach[C]//2023 IEEE 20th International Symposium on Biomedical Imaging (ISBI). IEEE, 2023:1-5.
https://ieeexplore.ieee.org/abstract/document/10230789
申请专利一:一种基于自监督 Graph-Transformer 的多模态大脑网络重要区域识别方法
顶刊NN发表论文:区分大脑状态——基于多段信号随机重组与交互式双向RNN
Zhang S, Shi E, Wu L, et al. Differentiating brain states via multi-clip random fragment strategy-based interactive bidirectional recurrent neural network[J]. Neural Networks, 2023, 165:1035-1049.
https://www.sciencedirect.com/science/article/abs/pii/S0893608023003520
2022年
会议MLMI 2023发表论文:两阶段多视图低秩稀疏子空间聚类方法探索脑功能与结构之间的关系
Zhang S, Kang Y, Yu S, et al. A Novel Two-Stage Multi-view Low-Rank Sparse Subspace Clustering Approach to Explore the Relationship Between Brain Function and Structure[C]//International Workshop on Machine Learning in Medical Imaging. Cham:Springer Nature Switzerland, 2022:191-200.
https://link.springer.com/chapter/10.1007/978-3-031-21014-3_20
期刊MedIA发表论文:一个可解释的用于表征和解释人脑状态的深度学习框架
Zhang S, Wang J, Yu S, et al. An explainable deep learning framework for characterizing and interpreting human brain states[J]. Medical Image Analysis, 2023, 83:102665.
https://www.sciencedirect.com/science/article/abs/pii/S1361841522002936
期刊Frontiers in Neuroscience发表论文:通过基于 DICCCOL 的多模态图神经网络区分早产儿和足月儿大脑,并标识相应的生物标志物
Zhang S, Wang R, Wang J, et al. Differentiate preterm and term infant brains and characterize the corresponding biomarkers via DICCCOL-based multi-modality graph neural networks[J]. Frontiers in Neuroscience, 2022, 16:951508.
https://www.frontiersin.org/articles/10.3389/fnins.2022.951508/full
期刊TNNLS发表论文:一种可解释且可推广的用于区分脑电图数据集上的人脑状态的循环神经网络方法
Zhang S, Wu L, Yu S, et al. An Explainable and Generalizable Recurrent Neural Network Approach for Differentiating Human Brain States on EEG Dataset[J]. IEEE Transactions on Neural Networks and Learning Systems, 2022.
https://ieeexplore.ieee.org/abstract/document/9940311
期刊Frontiers In Human Neuroscience发表论文:基于自然功能磁共振成像对大脑时空模式细粒度解释的混合学习框架
Yu S, Shi E, Wang R, et al. A hybrid learning framework for fine-grained interpretation of brain spatiotemporal patterns during naturalistic functional magnetic resonance imaging[J]. Frontiers in Human Neuroscience, 2022, 16:944543.
https://www.frontiersin.org/articles/10.3389/fnhum.2022.944543/full
期刊MedIA发表论文:基于 Gumbel-Softmax 的分层脑网络分解神经架构搜索
Pang T, Zhao S, Han J, et al. Gumbel-Softmax based Neural Architecture Search for Hierarchical Brain Networks Decomposition[J]. Medical Image Analysis, 2022, 82:102570.
https://www.sciencedirect.com/science/article/pii/S1361841522002110
期刊Human Brain Mapping发表论文:脑回峰:猕猴大脑发育过程中的新脑回标志
Zhang S, Chavoshnejad P, Li X, et al. Gyral peaks:novel gyral landmarks in developing macaque brains[J]. Human Brain Mapping, 2022, 43(15):4540-4555.
https://onlinelibrary.wiley.com/doi/full/10.1002/hbm.25971
期刊MedIA发表论文:通过多头引导注意力图神经网络(多头 GAGNN)对整体功能性大脑网络的时空模式进行建模
Yan J, Chen Y, Xiao Z, et al. Modeling spatio-temporal patterns of holistic functional brain networks via multi-head guided attention graph neural networks (Multi-Head GAGNNs)[J]. Medical Image Analysis, 2022, 80:102518.
https://www.sciencedirect.com/science/article/pii/S1361841522001657
期刊Chaos, Solitons & Fractals发表论文:基于DICCCOL的K最近界标检测方法,用于识别常见且一致的三铰链回旋折叠界标
Zhang S, Wang R, Han Z, et al. A DICCCOL-based K-nearest landmark detection method for identifying common and consistent 3-hinge gyral folding landmarks[J]. Chaos, Solitons & Fractals, 2022, 158:112018.
https://www.sciencedirect.com/science/article/abs/pii/S0960077922002284?via%3Dihub
期刊Cerebral Cortex发表论文:内在连接网络中脑回和脑沟之间功能差异的建模
Wang Q, Zhao S, He Z, et al. Modeling functional difference between gyri and sulci within intrinsic connectivity networks[J]. Cerebral Cortex, 2023, 33(4):933-947.
https://academic.oup.com/cercor/article-abstract/33/4/933/6553597
2021年
期刊Cerebral Cortex发表论文:脑回铰链在皮质网络中具有最高的成本和最高的通信能力
He Z, Du L, Huang Y, et al. Gyral hinges account for the highest cost and the highest communication capacity in a corticocortical network[J]. Cerebral Cortex, 2022, 32(16):3359-3376.
https://academic.oup.com/cercor/article-abstract/32/16/3359/6454661
期刊Frontiers in Neuroscience发表论文:通过典型相关分析和局部保留投影对早产儿和足月婴儿大脑之间的功能和结构连接体进行联合分析
Zhang S, He Z, Du L, et al. Joint analysis of functional and structural connectomes between preterm and term infant brains via canonical correlation analysis with locality preserving projection[J]. Frontiers in Neuroscience, 2021, 15:724391.
https://www.frontiersin.org/articles/10.3389/fnins.2021.724391/full
会议MLMI 2021发表论文:通过解剖引导的时空图卷积网络探索跨任务域的脑回-脑沟功能连接差异
Jiang M, Yang S, Zhao Z, et al. Exploring Gyro-Sulcal Functional Connectivity Differences Across Task Domains via Anatomy-Guided Spatio-Temporal Graph Convolutional Networks[C]//Machine Learning in Medical Imaging:12th International Workshop, MLMI 2021, Held in Conjunction with MICCAI 2021, Strasbourg, France, September 27, 2021, Proceedings 12. Springer International Publishing, 2021:130-139.
https://link.springer.com/chapter/10.1007/978-3-030-87589-3_14