顶会MICCAI 2023发表论文:基于自监督Graph-Transformer探索大脑功能结构连接体骨架
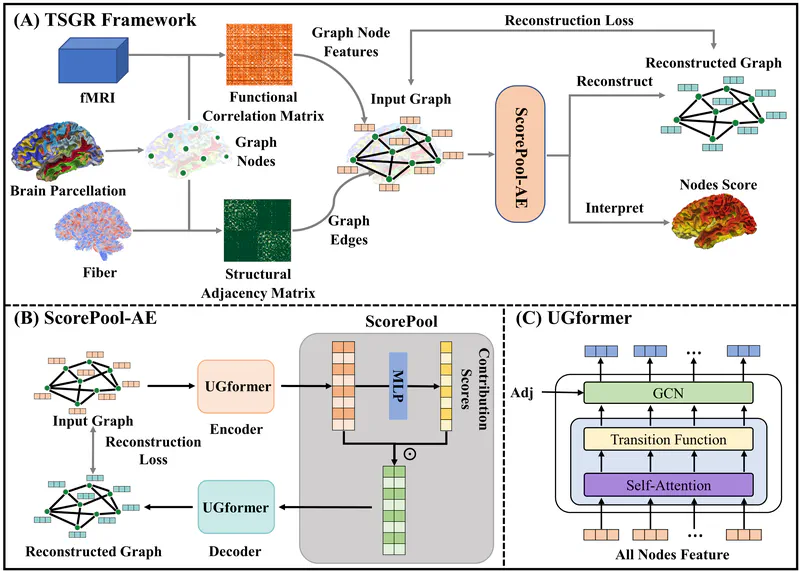
论文 “Exploring Brain Function-Structure Connectome Skeleton via Self-Supervised Graph-Transformer Approach” 被会议MICCAI 2023接收
时间:2023-06-24
关键词:脑影像人工智能,文章接收
硕士研究生康艳晴在MICCAI 2023上面发表题为 “Exploring Brain Function-Structure Connectome Skeleton via Self-Supervised Graph-Transformer Approach” 的研究生文章,通讯作者为张枢教授。
Understanding the relationship between brain functional connectivity and structural connectivity is important in the field of brain imaging, and it can help us better comprehend the working mechanisms of the brain. Much effort has been made on this issue, but it is still far from satisfactory. The brain transmits information through a network architecture, which means that the regions and connections of the brain are significant. The main difficulties with this issue are currently at least two aspects. On the one hand, the importance of different brain regions in structural and functional integration has not been fully addressed; on the other hand, the connectome skeleton of the brain, plays the role in common and key connections in the brain network, has not been clearly studied. To alleviate the above problems, this paper proposes a transformer-based self-supervised graph reconstruction framework (TSGR). The framework uses the graph neural network (GNN) to fuse functional and structural information of the brain, reconstructs the brain graph through a self-supervised model and identifies the regions that are important to the reconstruction task. These regions are considered as key connectome regions which play an essential role in the communication connectivity of the brain network. Based on key brain regions, the connectome skeleton can be obtained. Experimental results demonstrate the effectiveness of the proposed method, which obtains key regions and connectome skeleton in the brain network. This provides a new angle of view to explore the relationship between brain function and structure. Our code is available at https://github.com/kang105/TSGR.
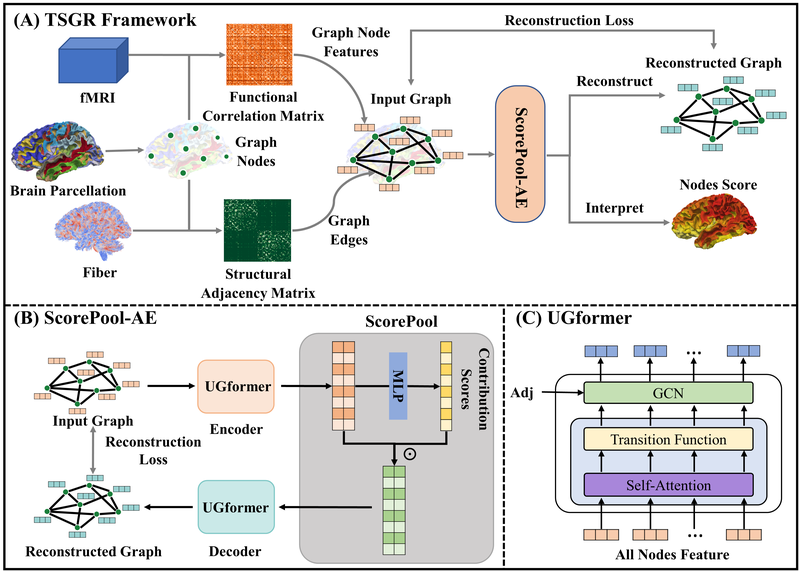