期刊CIBM发表论文:通过自监督graph transformer识别大脑网络中的关键节点
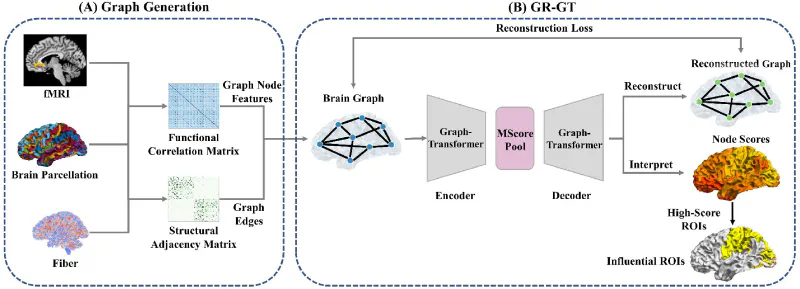
论文 “Identifying influential nodes in brain networks via self-supervised graph-transformer” 在期刊《Computers in Biology and Medicine》 (CIBM) 发表
时间:2024-12-27
关键词:脑影像人工智能,文章接收
硕士研究生康艳晴在《Computers in Biology and Medicine》上发表题为 “Identifying influential nodes in brain networks via self-supervised graph-transformer” 的研究文章,通讯作者为张枢教授。
Studying influential nodes (I-nodes) in brain networks is of great significance in the field of brain imaging. Most existing studies consider brain connectivity hubs as I-nodes such as the regions of high centrality or rich-club organization. However, this approach relies heavily on prior knowledge from graph theory, which may overlook the intrinsic characteristics of the brain network, especially when its architecture is not fully understood. In contrast, self-supervised deep learning dispenses with manual features, allowing it to learn meaningful representations directly from the data. This approach enables the exploration of I-nodes for brain networks, which is also lacking in current studies. This paper proposes a Self-Supervised Graph Reconstruction framework based on Graph-Transformer (SSGR-GT) to identify I-nodes, which has three main characteristics. First, as a self-supervised model, SSGR-GT extracts the importance of brain nodes to the reconstruction. Second, SSGR-GT uses Graph-Transformer, which is well-suited for extracting features from brain graphs, combining both local and global characteristics. Third, multimodal analysis of I-nodes uses graph-based fusion technology, combining functional and structural brain information. The I-nodes we obtained are distributed in critical areas such as the superior frontal lobe, lateral parietal lobe, and lateral occipital lobe, with a total of 56 identified across different experiments. These I-nodes are involved in more brain networks than other regions, have longer fiber connections, and occupy more central positions in structural connectivity. They also exhibit strong connectivity and high node efficiency in both functional and structural networks. Furthermore, there is a significant overlap between the I-nodes and both the structural and functional rich-club. Experimental results verify the effectiveness of the proposed method, and I-nodes are obtained and discussed. These findings enhance our understanding of the I-nodes within the brain network, and provide new insights for future research in further understanding the brain working mechanisms.
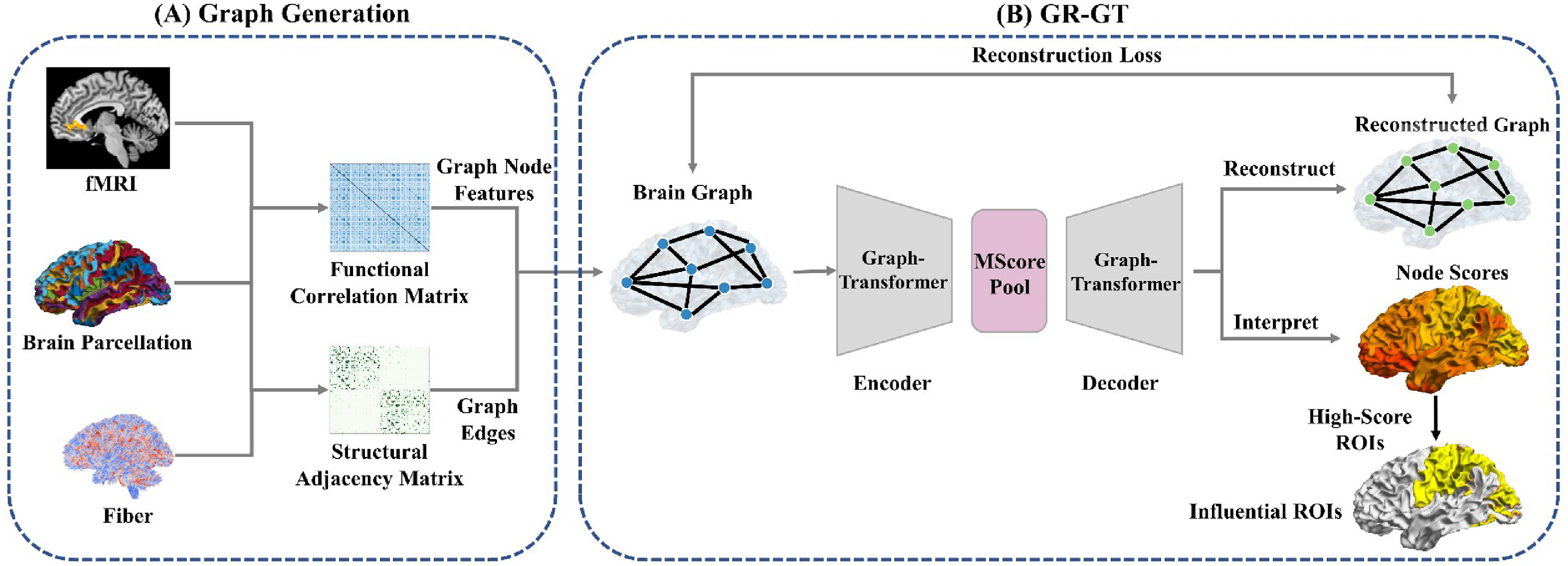
参考文献
Kang Y, Zhu D, Zhang H, et al. Identifying influential nodes in brain networks via self-supervised graph-transformer[J]. Computers in Biology and Medicine, 2025, 186: 109629.
https://www.sciencedirect.com/science/article/pii/S0010482524017141